Synthetic extinct generations methods
Description of method
Bennett and Horiuchi (1981, 1984) generalized the Preston and Coale method for estimating the completeness of the reporting of deaths relative to an estimate of the population, into what has eventually become known as the Synthetic Extinct Generations (SEG) method. Basically these methods make use of the observation that, in a closed population, the number of people of a given age, a, alive at a point in time must equal the number of people who will die from that age forward. The key insight on which SEG methods are based is that the future stream of deaths of a cohort can be replaced by current deaths at each age above a if the effects of future age-specific population growth can be taken into account. The simplest example is a stationary (life table) population, in which future deaths above age a will be equal to current deaths above that age. Somewhat more complex is the case where the population is stable (i.e. a population with an unchanging adult age distribution growing at a constant rate, r, each year) and closed to migration. In this case, if the reported data are accurate, the number of deaths at age x, t years in the future, will equal the number of deaths at age x currently, multiplied by . This is the model underlying the Preston and Coale method.
In the more general case where the population is not stable, an equivalent relationship exists if one replaces by where r(y¸t) represent the growth rate of the population aged y at time t.
If the deaths reported at time t can be assumed to be reported to the same extent, c, at every adult age, then the estimate of the future number of cohort deaths will be underestimated to the same extent. Thus, it is possible to estimate the completeness of reporting of deaths by dividing the sum of the estimates of future cohort deaths derived from the number of deaths at any date by the population at the same date. Mortality rates can then be estimated by dividing the numbers of deaths reported in each adult age group by c and then dividing these numbers by an estimate of the population exposed to risk based on the population used to estimate the partial birth and death rates.
Data requirements and assumptions
Tabulations of data required
- Number of women (men), by five-year age group, and for open age interval A+ (with A as high as possible), at two points in time, typically from the results of two censuses. (See the caveat below concerning the use of surveys rather than censuses.)
- Number of deaths of women (men), by five-year age group, and for open age interval A+, over the period between the two censuses or surveys
Important assumptions
- The coverage of each census is the same for all ages.
- The completeness of reporting of deaths is the same for all ages above some minimum age (usually age 15).
- The population is closed to migration. Although the method can be adapted to allow for migration, accurate enough estimates of the net numbers of migrants to do so seldom exist. For national populations, net migration is often low enough to ignore, but for situations where migration is significant one needs to take this into account when interpreting results and deciding on an estimate of completeness.
Preparatory work and preliminary investigations
Before applying this method, you should examine the quality of the data in at least the following dimensions:
- age structure of the population;
- sex structure of the population;
- age structure of the deaths; and
- sex structure of the deaths.
If the reported deaths are for a period other than that between the censuses the numbers that would have been reported in the intercensal period need to be estimated. If one has annual vital registration data, this adjustment involves apportioning deaths in the first and last year of the period. If one has deaths reported by households the year before the dates of each of the first and second censuses, one has to estimate the numbers of deaths by interpolating between these estimates for the intercensal period (using the Estimating deaths spreadsheet).
The SEG methodology uses age-specific population growth rates in its calculations. If the completeness of census enumeration varies from one census to the next by a proportionately constant amount at all ages, such growth rates will be biased by a fixed amount, delta. The Generalized Growth Balance methodology explicitly estimates this bias. The SEG methodology does not explicitly estimate delta, but non-zero values of delta result in a linear trend in estimates of completeness of death recording with age. Thus delta can be estimated iteratively by finding the value that produces coverage estimates that are constant by age between selected lower and upper age limits.
Caveats and warnings
In applying this method, analysts must take particular care with the following.
- The interpretation and estimating processes need to take into account the source of death data (vital registration, reported by households in censuses, or recorded in hospitals) as explained below. Biases associated with the source of death data tend to have more impact on the estimate of completeness from the Synthetic Extinct Generations method than on the Generalized Growth Balance method.
- If applying the method to sub-national geographic areas, the issue of migration typically becomes a greater concern.
- Deciding the age range which is to be used to determine delta (the estimate of the coverage of one census relative to the other). Issues here are whether the best estimate of delta is the intercept determined as a result of applying the Generalized Growth Balance method to the same data (which would be the case, for example, if completeness of reporting of deaths was thought to decrease after retirement, for reasons explained below), and whether to exclude ages below 30 or 35 because of the impact of migration which has not been allowed for specifically.
- Deciding on the age range to use for determining the estimate of completeness. Typically this range might exclude young adults if there is significant unaccounted-for migration, or the elderly if the results suggest that a lower proportion of their deaths are reported than of deaths of younger adults or if age misreporting of the elderly appears to affect ages of the living and the dead differently.
- Ensuring that the solver routine in Excel has run satisfactorily (i.e. has produced a sensible result). Occasionally Solver offers a solution which is manifestly too low. In such situations it is best to adjust delta manually in the right direction and apply Solver to this new starting value.
- Ensuring that the estimate of life expectancy at the age of the open interval is reasonable. Often data on older people are scanty and particularly prone to errors. Thus estimates of life expectancy based on these data can be implausible (usually over-estimating life expectancy). The higher the age of the open interval, the lower the impact of any error.
- If completeness appears to be less than 60 per cent, then the uncertainty is large and this should be taken into account when interpreting the results.
- It is tempting in a situation in which census data on the age distribution of the population and household deaths are available for only one census, to combine this with data on the age distribution of the population from a sample survey at some earlier or later date. However, for reasons that have not been adequately researched, such a combination of data sources rarely gives satisfactory results.
Application of method
Although technically one could apply this method to data in single year age categories, the data one typically works with are subject to age misstatement, so in practice one usually works with data grouped into five-year age groups. For convenience, since most data are published in this format, the spreadsheet is set up to work with data in the standard five-year groupings. However, as Blacker (1988) has shown, if this grouping fails to remove the effect of digit preference, the method should be adapted to work with an alternative five-year grouping of ages centred on, rather than starting with, ages at which heaping occurs.
Step 1: If not readily available, estimate the number of deaths reported in the period between the dates of the two estimates of the population
In the case where one has annual vital registration data, this adjustment involves apportioning deaths in the first and last year of the period to the parts of the year before and after the mean dates of fieldwork of the two inquiries. Unless the age pattern of deaths is changing very rapidly, this will have no effect on the results.
If one lacks data on the number of deaths between the two inquiries but this interval falls between two periods for which one does have such estimates (for example, because each inquiry included a question about deaths in the household during the previous year), one can make use of the Estimating deaths spreadsheet. This spreadsheet estimates the number of deaths between two points in time given estimates of deaths over two other periods. To use this spreadsheet, you need the number of deaths divided into five-year age groups for two periods (periods 1 and 2), the start and end dates for each of these periods, and the start date and end date of the period for which one wishes to estimate the number of deaths.
Step 2: Estimate the growth rates adjusting for migration and differential census coverage
Age-specific growth rates adjusting for migration and differential census coverage are estimated from the two census populations and the numbers of migrants over the intercensal period by age group as follows:
where is the population aged between x and x + 5 at time t, 5NMx is the net number of migrants (in- less out-migrants) aged between x and x + 5, and t1 and t2 are the times of the two censuses. Delta is the correction for the completeness of one census relative to the other. It is either set equal to the estimate from the Generalized Growth Balance method, or solved for iteratively as explained below.
Step 3: Estimate the life expectancy at age A and at each five-year age intervals down to 65
This can be done in one of several ways.
- Use estimates from an independent source, if reliable estimates are available. Possible sources would be estimates produced by previous research or from population projections such as the World Population Prospects (UN Population Division 2011).
- Use the estimates derived from the data after applying the Generalized Growth Balance method. The workbook implementing that method produces such estimates as part of the output.
- Use the ratio of the reported deaths in the age group 10 to 39 last birthday to those in the age group 40 to 59 last birthday (30D10/20D40) to determine (by comparison) a level of the West model life table, from which estimates of life expectancy can be read. These estimates are included as part of the workbook implementing this method. Unfortunately, since the West model life table does not reflect mortality resulting from HIV/AIDS, this approach is unsuitable for countries that have significant numbers of AIDS deaths.
- Solve for the life expectancy iteratively by starting with a reasonable guess such as those estimated from the West table (although in some cases this may not work in countries with significant numbers of AIDS deaths) or from an independent source. Then estimate completeness (as described below), copy the life expectancies based on this level of completeness from the Life expectancies spreadsheet of the associated workbook, paste the values of these into the Method spreadsheet of the associated workbook and re-estimate completeness. Repeat if necessary until the change to life expectancies is no longer significant. Unfortunately, if there are reasons for suspecting that, even after correcting the rates for incompleteness, mortality is underestimated at the older ages (for example, if there is significant age exaggeration, or relatively higher incompleteness at the older ages) then this approach will overestimate the life expectancies and hence overestimate the overall level of completeness of reporting.
Step 4: Estimate the number of people who turned x, and the number aged x to x+4 last birthday, from the reported deaths
The number of people who turned x during the period over which the deaths were reported is estimated from the reported deaths as follows:
and
where A is the age at the start of the open interval, nrx is the annual population growth rate in the age group x to x+n last birthday, and eA is the life expectancy at age A.
The number of people aged x to x + 4 last birthday during the period over which the deaths were reported is estimated from the numbers who turned x in five-year steps as follows:
Step 5: Estimate the number of people aged x to x + 4 last birthday during the period between the two censuses, from the census populations
The number of people aged x to x + 4 during the period over which the deaths are reported is estimated from the census populations by multiplying the geometric mean of the numbers in that age group in the two census populations by the length of the period between the two censuses (measured in years) as follows:
Step 6: Calculate the ratios of the estimates derived from deaths to those derived from the census populations
Two sets of ratios of the estimates derived from the deaths to those derived from the census population are calculated. The first is the ratios in quinquennial age groups, which are calculated directly. The second is the ratios of the numbers from age x to that age of the open interval, A, with the numbers of people who turned x to A-1 during the period being calculated as the aggregate of the numbers in five-year age groups between ages x and A-5. In other words,
Step 7: Estimate the completeness of reporting of deaths
In order to determine the level of completeness of reporting one first needs to decide if the growth rates need to be corrected for relative completeness of the population censuses. The interpretation of the plots of the ratios is discussed in more detail below. However, essentially the amount by which the growth rates need to be corrected (delta) for relative completeness of the census populations is identified as the amount which produces the most level set of ratios by age. The Method spreadsheet is set up so that Solver (Data, Solver, Solve) will find the value of delta that minimizes the absolute deviation from the mean of the ratios over the age range specified by the user.
It is suggested that the intercept, a, from the application of the Generalized Growth Balance method to the same data be used as an initial estimate of delta. If this estimate of delta produces a level series of ratios across adult ages but with significant curvature downward at the older ages, this could indicate a fall off of completeness at the older ages. This might be the case if, for example, people retired from urban areas to rural areas, where completeness of registration was lower, or there was a drop off in reporting of deaths by households due to disintegration of some households on the death of a member. In such a situation it is important not to set delta to produce a level set of ratios, but rather to use the initial value.
If one is solving for both delta and the life expectancies iteratively, the values of life expectancies will need to be pasted from the Life expectancies spreadsheet into the Method spreadsheet and a new estimate of delta set. This process may need to be repeated two or three times, until there is no change in the life expectancies.
Finally, one decides on the age range of ratios to be used to determine the completeness. If there is a significant curvature upward at the older ages, this probably indicates age exaggeration, particularly for deaths, and one needs to try and identify an age for the open interval below which the age exaggeration is not significant. If completeness drops off at ages below 35, this could indicate unaccounted for out-migration. If this is suspected then one should exclude these ages from determining delta or completeness.
Completeness is estimated from the quinquennial age group-specific ratios. In order to produce a robust estimate it is calculated as the sum of 50 per cent of the median plus 25 per cent of each of the 75th and 25th percentile of these ratios.
Step 8: Estimate mortality rates adjusted for incompleteness of reporting of deaths
In order to compute mortality rates one needs first to correct the census population for relative under enumeration. This is achieved by multiplying the numbers from the first census by , and the numbers from the second census by 1, if delta is less than zero, and the reverse if delta >0.
The adjusted person-years of exposure, PYLa(x,5), are estimated by multiplying the geometric average of the corrected populations by the length of the period between the censuses (in years) as follows:
Next, one needs to adjust the number of deaths for incompleteness by dividing the reported number of deaths by the estimate of completeness, c, and dividing this by PYLa(x,5) to produce mortality rates adjusted for the incompleteness of the reporting of deaths as follows:
If it is decided that completeness fell after retirement then the estimates of the rates at these ages might be improved by replacing c by the age group-specific estimates of completeness at these ages. There is an option in the spreadsheet to implement this.
Note that technically one could drop the adjustment for under-coverage of one census relative to the other and still get the same estimates of the mortality rates since the same adjustment is made to both the numerator and the denominator. However, in that case the estimate of completeness is relative to the average of the census populations ignoring the fact that one is undercounted relative to the other.
Step 9: Smooth using relational logit model life table
Because the age-specific rates can be quite erratic they need to be graduated (smoothed). This can be achieved by fitting a Brass relational logit function to a sex-specific standard life table which is considered to have the same shape as that generated by the mortality rates of the population being investigated.
The accompanying workbook contains a spreadsheet that allows one to produce a smooth set of mortality rates by using a relational logit model fitted to the life table generated by the adjusted mortality rates. The user can choose between the standard from the General family of United Nations model life tables or one from any of the four families of Princeton model life tables. The logit transforms of these tables together with a model life table of a population experiencing an AIDS epidemic (Timæus 2007) appear in the Models spreadsheet. This spreadsheet also allows the user to input logit transforms of an alternative life table if there is reason to assume that it has a similar pattern of adult mortality to that of the population being studied.
In order to fit the model, probabilities of people aged x dying in the next 5 years, 5qx, are estimated from the adjusted rates of mortality as follows:
From this the life table with a radix of l5 = 1 is calculated as follows:
The coefficients, α and β are determined by fitting the relational logit model as follows:
where and superscript ‘s' designates values based on a standard life table.
The fitted life table is then generated from the standard life table using the coefficients α and β as follows:
and
The smoothed mortality rates are derived from this life table as follows:
and
where
i.e.
and ω is the age above which the life table has no more survivors.
The life expectancies, which are of particular interest if one wants to estimate the life expectancies at the older ages iteratively, are derived as follows:
Worked example
This example uses data on the numbers of males in the population from the South African Census in 2001 and the Community Survey in 2007, on number of deaths from vital registration for the years 2001 to 2007, and on the net number of migrants estimated from the change in foreign-born counted in the two surveys, less an estimate of the number of South Africans who emigrated between the two surveys. The example appears in the SEG South_Africa_males workbook.
Step 1: If not readily available, estimate the number of deaths reported in the period between the dates of the two estimates of the population
The registered deaths for the years 2001 to 2007 for South African males are given in Table 1.
Table 1 Calculation of deaths between census dates, South African males, 2001-2007
Age |
2001 |
2002-2006 |
2007 |
Total between censuses |
0-4 |
29,005 |
186,346 |
40,314 |
197,912 |
5-9 |
2,118 |
14,733 |
2,854 |
15,566 |
10-14 |
1,745 |
10,535 |
2,233 |
11,207 |
15-19 |
4,470 |
23,857 |
4,860 |
25,473 |
20-24 |
8,931 |
51,588 |
10,875 |
54,960 |
25-29 |
16,834 |
96,705 |
18,405 |
102,802 |
30-34 |
20,892 |
137,355 |
28,245 |
145,588 |
35-39 |
21,068 |
137,502 |
29,258 |
145,900 |
40-44 |
19,322 |
128,217 |
26,973 |
135,936 |
45-49 |
17,881 |
113,891 |
24,761 |
121,010 |
50-54 |
16,883 |
104,508 |
22,790 |
111,157 |
55-59 |
14,544 |
90,919 |
21,317 |
96,854 |
60-64 |
15,097 |
84,351 |
17,410 |
89,930 |
65-69 |
13,011 |
77,680 |
17,878 |
82,843 |
70-74 |
14,035 |
68,147 |
13,771 |
73,036 |
75-79 |
10,846 |
59,859 |
12,534 |
63,871 |
80-84 |
9,161 |
44,986 |
8,872 |
48,163 |
85+ |
7,602 |
43,233 |
10,009 |
46,196 |
The reference time for the Census in 2001 was midnight between 9 and 10 October 2001. The Community Survey took place over a number of weeks in February so we can assume a reference time of midnight between 14 and 15 February 2007. Thus if we assume deaths occur uniformly over the respective calendar years we can apportion the deaths in 2001 and 2007 and add these to the total for the years 2002 to 2006 to get the total number of deaths between the two estimates of the population. For example, for the age group 20-24 the number is calculated as follows:
Step 2: Estimate the growth rates adjusting for migration and differential census coverage
Age-specific growth rates less the net in-migration rate and adjusted for differential census coverage appear in column 6 of Table 2. They are calculated for the 20 to 25 age group, for example, using the populations given in columns 2 and 3, the net in-migration given in column 5 of Table 2 and delta (estimated below) as follows:
where 5.3541 is the time between the census and survey calculated using the YEARFRAC functions in Excel.
Table 2 Growth rates and estimate of the numbers who turned x and the numbers aged between x and x+5 derived from the numbers of deaths, South African males, 2001-2007
Age |
5Nx(t1) |
5Nx(t2) |
5Dx |
5NMx |
5rx |
Est Nx |
Est 5Nx |
|
|
0 |
2,223,006 |
2,505,744 |
197,912 |
10,605 |
0.0168 |
|
|
||
5 |
2,425,066 |
2,560,642 |
15,566 |
2,848 |
0.0053 |
2,304,653 |
11,334,968 |
||
10 |
2,518,985 |
2,452,339 |
11,207 |
5,153 |
-0.0101 |
2,229,335 |
11,405,753 |
||
15 |
2,453,156 |
2,553,293 |
25,473 |
16,574 |
0.0016 |
2,332,967 |
11,556,063 |
||
20 |
2,099,417 |
2,362,519 |
54,960 |
14,803 |
0.0161 |
2,289,459 |
10,871,687 |
||
25 |
1,899,275 |
2,033,165 |
102,802 |
4,714 |
0.0076 |
2,059,216 |
9,851,950 |
||
30 |
1,594,624 |
1,875,483 |
145,588 |
13,331 |
0.0242 |
1,881,564 |
8,529,425 |
||
35 |
1,441,657 |
1,548,185 |
145,900 |
9,693 |
0.0074 |
1,530,206 |
7,153,512 |
||
40 |
1,233,813 |
1,306,900 |
135,936 |
7,464 |
0.0050 |
1,331,199 |
6,238,580 |
||
45 |
967,744 |
1,104,294 |
121,010 |
8,719 |
0.0184 |
1,164,233 |
5,276,384 |
||
50 |
769,627 |
888,042 |
111,157 |
9,413 |
0.0199 |
946,320 |
4,242,847 |
||
55 |
552,402 |
708,812 |
96,854 |
4,640 |
0.0405 |
750,818 |
3,191,145 |
||
60 |
444,592 |
491,871 |
89,930 |
5,081 |
0.0122 |
525,640 |
2,332,526 |
||
65 |
304,835 |
394,305 |
82,843 |
4,922 |
0.0407 |
407,371 |
1,662,114 |
||
70 |
232,604 |
241,976 |
73,036 |
4,334 |
-0.0007 |
257,475 |
1,106,744 |
||
75 |
136,466 |
163,112 |
63,871 |
2,980 |
0.0249 |
185,223 |
721,856 |
||
80 |
90,856 |
87,698 |
48,163 |
1,662 |
-0.0148 |
103,519 |
412,486 |
||
85 |
45,920 |
70,299 |
46,196 |
2,009 |
0.0683 |
61,475 |
|
Step 3: Estimate the life expectancy at age A and five-year age intervals down to 65
The estimates derived after applying the Generalized Growth Balance method to the same data are as shown in column 2 of Table 3.
The ratio of the reported deaths in the age group 10 to 39 last birthday to those in the age group 40 to 59 last birthday from column 4 of Table 2 is
The life expectancies of the male Princeton West model life table which corresponds to this are determined (from the table in the Life expectancies spreadsheet of the workbook) by interpolation and are shown in column 3 of Table 3. For example for age 65:
Solving for the life expectancy and delta iteratively by starting with the estimates from the West table produces an estimate of delta (as explained in more detail below) of ‑0.0066 and the final estimates of life expectancy which appear in column 4 of Table 3.
Table 3 Life expectancies from different sources, South African males 2001-2007
x |
Generalized Growth Balance |
Princeton West |
Iterative estimates |
Delta fixed to GGB estimate |
65 |
11.7 |
9.45 |
11.6 |
11.7 |
70 |
9.4 |
7.37 |
9.3 |
9.4 |
75 |
7.4 |
5.55 |
7.3 |
7.4 |
80 |
5.7 |
4.06 |
5.6 |
5.7 |
85 |
4.4 |
2.90 |
4.3 |
4.4 |
Since the prevalence of HIV/AIDS was high in South Africa one cannot use the estimates derived from the West life tables given in the Life expectancies spreadsheet of the workbook in estimating the completeness of reporting of deaths. In addition, since – discussed below – it appears that completeness could be falling with age for ages above age 55, the iterative estimates may not be ideal. Thus for this example, delta is set equal to the intercept, a, from the application of the Generalized Growth Balance method to the same data, and the life expectancies are as appear in column 5 of Table 3.
Step 4: Estimate the number of people who turned x, and the number aged x to x + 4 last birthday, from the reported deaths
The number of people who turned x during the period between the two censuses as estimated from the numbers of deaths using an open interval of 85+, growth rates from column 6 of Table 2 and the estimate of life expectancy at age 85 of 4.347 given in the fifth column of Table 3, is as shown in column 7 of Table 2. For example, the estimate of the number of people who turned 80 in the period between the censuses is calculated as follows, using the growth rate for the population in open interval 85+ of 0.0638 and the growth rate for the population aged between 80 and 85 of -0.0148, is:
The number of people aged x to x+4 last birthdays during the period between the two censuses, estimated from the reported deaths is given in column 8 of Table 2. For example, the number who turned 20 to 24 last birthday is calculated as follows:
Step 5: Estimate the number of people aged x to x+4 last birthday during the period between the censuses, from the census populations
The number of people aged between x and x + 5 during the period between the two censuses appears in column 2 of Table 4 and is calculated for the 20 to 24 age group, for example, using the populations given in columns 2 and 3 of Table 2 and the time between the two censuses, as follows:
Table 4 The number aged x to x+4 last birthday estimated from the census population and the ratios of the estimates derived from the numbers of deaths to this, South African males, 2001-2007
Age |
Obs 5Nx |
c: 5Nx |
c: A-xNx |
0 |
12,636,377 |
|
|
5 |
13,341,976 |
0.8496 |
0.8981 |
10 |
13,307,209 |
0.8571 |
0.9050 |
15 |
13,399,755 |
0.8624 |
0.9130 |
20 |
11,923,972 |
0.9118 |
0.9231 |
25 |
10,521,174 |
0.9364 |
0.9256 |
30 |
9,259,118 |
0.9212 |
0.9230 |
35 |
7,998,828 |
0.8943 |
0.9235 |
40 |
6,798,761 |
0.9176 |
0.9322 |
45 |
5,534,858 |
0.9533 |
0.9371 |
50 |
4,426,301 |
0.9586 |
0.9310 |
55 |
3,350,250 |
0.9525 |
0.9191 |
60 |
2,503,746 |
0.9316 |
0.9028 |
65 |
1,856,232 |
0.8954 |
0.8865 |
70 |
1,270,220 |
0.8713 |
0.8799 |
75 |
798,803 |
0.9037 |
0.8885 |
80 |
477,921 |
0.8631 |
0.8631 |
Step 6: Calculate the ratios of the estimates derived from deaths to those derived from the census populations
The ratios of the numbers of people aged between x and x + 5 during the period between the censuses estimated from the reported deaths (column 8 of Table 2) to those estimated from the censuses (column 2 of Table 4) are given in columns 3 and 4 of Table 4. Examples of these calculations for age 65 are as follows:
Step 7: Estimate the completeness of reporting of deaths
Setting delta to the intercept of the application of the Generalized Growth Balance method produces a series of ratios which, although reasonably level, appears to fall off with increasing age from about age 50 (see from Figure 1). Thus for this example Solver was not used to estimate delta.
Completeness was estimated from the ratios in the age range 25 to 64. This was done to avoid, to some extent, biasing the estimate downwards due to the falling off of the ratios at the extreme ages although the method of determining the estimate is fairly robust to fluctuations at individual ages. This produced an estimate of completeness of 94 per cent as follows:
where 0.9340 is the median, 0.9203 the 25th percentile and 0.9527 the 75th percentile of the ratios in column 3 of Table 4 between ages 25 and 65.
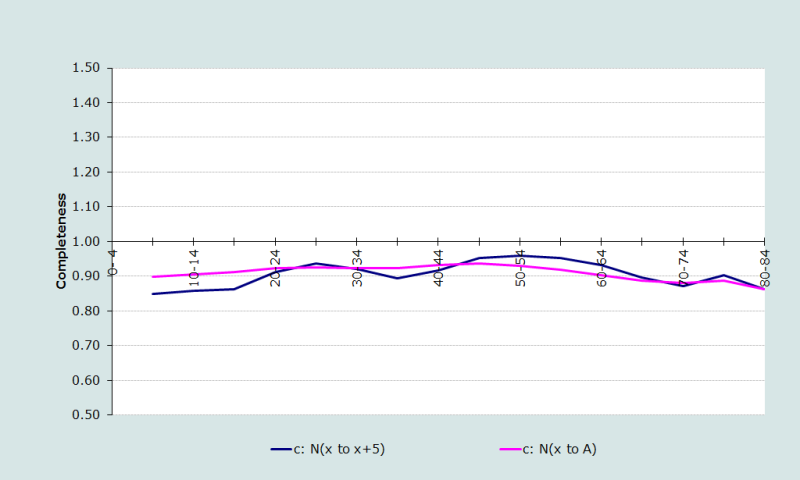
Step 8: Estimate mortality rates adjusted for incompleteness of reporting of deaths
The adjusted population as at the first census date which appears in column 2 of Table 5 is the enumerated population given in column 2 of Table 2 multiplied by exp(‑(‑0.00467)x5.3541) since delta is less than 0. For example the adjusted population for age 20 is
The adjusted population at the second census date which appears in column 3 of Table 5 is the enumerated population given in column 3 of Table 2 since delta is less than 0.
Next the deaths are adjusted for incompleteness by dividing the number of reported deaths in each age group shown in column 4 of Table 2 by the estimate of completeness. These numbers are shown in column 4 of Table 5. For example, for age 20 the number is derived from the number of reported deaths, 54 960, as follows:
As it appears that completeness may have declined at the older ages, the option to use age-specific completeness above age 65 is chosen. Thus, for example, the number of deaths between 70 and 75 corrected for incompleteness is calculated as follows:
The adjusted person-years of life lived (column 5 of Table 5) is the geometric average of the populations in columns 2 and 3 of Table 5 multiplied by the length (in years) of the period between the censuses, which in this case is 5.3541 years. For age 20 this is
The mortality rates adjusted for incompleteness of reporting of deaths (column 6 of Table 5) are derived by dividing the adjusted deaths by the adjusted person-years of life lived. For example, for the 20-24 age group the adjusted rate is calculated as follows:
Table 5 Calculation of adjusted mortality rates, South African males, 2001-2007
Age |
Adjusted 5Nx(t1) |
Adjusted 5Nx(t2) |
Adjusted 5Dx |
Adjusted PYL(x,5) |
Adjusted 5mx |
0 |
|
|
|
|
|
5 |
2,486,532 |
2,560,642 |
16,644 |
13,510,001 |
0.0012 |
10 |
2,582,831 |
2,452,339 |
11,983 |
13,474,797 |
0.0009 |
15 |
2,515,334 |
2,553,293 |
27,236 |
13,568,508 |
0.0020 |
20 |
2,152,629 |
2,362,519 |
58,764 |
12,074,140 |
0.0049 |
25 |
1,947,414 |
2,033,165 |
109,919 |
10,653,675 |
0.0103 |
30 |
1,635,041 |
1,875,483 |
155,667 |
9,375,725 |
0.0166 |
35 |
1,478,197 |
1,548,185 |
156,001 |
8,099,564 |
0.0193 |
40 |
1,265,085 |
1,306,900 |
145,347 |
6,884,383 |
0.0211 |
45 |
992,273 |
1,104,294 |
129,387 |
5,604,563 |
0.0231 |
50 |
789,134 |
888,042 |
118,852 |
4,482,045 |
0.0265 |
55 |
566,403 |
708,812 |
103,560 |
3,392,442 |
0.0305 |
60 |
455,861 |
491,871 |
96,156 |
2,535,277 |
0.0379 |
65 |
312,561 |
394,305 |
92,518 |
1,879,609 |
0.0492 |
70 |
238,500 |
241,976 |
83,824 |
1,286,217 |
0.0652 |
75 |
139,925 |
163,112 |
70,679 |
808,863 |
0.0874 |
80 |
93,159 |
87,698 |
55,803 |
483,940 |
0.1153 |
85 |
47,084 |
70,299 |
53,524 |
308,032 |
0.1738 |
Step 9: Smooth using relational logit model life table
Estimates of probabilities of people aged x dying in the next 5 years, 5qx, estimated from the adjusted rates of mortality which appear in column 6 of Table 5, are shown in the second column of Table 6. For example, the probability of a 20-year old woman dying before reaching age 25 is calculated as follows:
The life table proportions of five-year olds alive at age x+5, estimated from the proportion alive at age x using these values, appear in column 3 of Table 6. For example the proportion alive at age 25 is calculated as follows:
Table 6 Calculation of smoothed mortality rates using a relational logit model life table, South African males, 2001-2007
Age |
5qx |
lx/l5 |
Obs. Y(x) |
AIDS Cdn. ls(x) |
Cdn. Ys(x) |
Fitted Y(x) |
Fitted l(x) |
T(x) |
e(x) |
Smooth 5mx |
0 |
|
|
|
|
|
|
|
|
|
|
5 |
0.0061 |
1 |
|
1.0000 |
|
|
1 |
51.206 |
51.2 |
0.0030 |
10 |
0.0044 |
0.9939 |
-2.5433 |
0.9785 |
-1.9081 |
-2.0984 |
0.9852 |
46.243 |
46.9 |
0.0028 |
15 |
0.0100 |
0.9894 |
-2.2705 |
0.9632 |
-1.6326 |
-1.7676 |
0.9717 |
41.351 |
42.6 |
0.0024 |
20 |
0.0240 |
0.9796 |
-1.9350 |
0.9512 |
-1.4853 |
-1.5907 |
0.9601 |
36.521 |
38.0 |
0.0041 |
25 |
0.0503 |
0.9560 |
-1.5395 |
0.9324 |
-1.3120 |
-1.3827 |
0.9408 |
31.769 |
33.8 |
0.0086 |
30 |
0.0797 |
0.9079 |
-1.1444 |
0.8969 |
-1.0818 |
-1.1062 |
0.9014 |
27.164 |
30.1 |
0.0152 |
35 |
0.0919 |
0.8356 |
-0.8128 |
0.8420 |
-0.8365 |
-0.8116 |
0.8352 |
22.822 |
27.3 |
0.0200 |
40 |
0.1003 |
0.7588 |
-0.5731 |
0.7794 |
-0.6311 |
-0.5650 |
0.7559 |
18.845 |
24.9 |
0.0235 |
45 |
0.1091 |
0.6827 |
-0.3831 |
0.7148 |
-0.4593 |
-0.3588 |
0.6721 |
15.275 |
22.7 |
0.0239 |
50 |
0.1243 |
0.6082 |
-0.2199 |
0.6560 |
-0.3228 |
-0.1948 |
0.5962 |
12.104 |
20.3 |
0.0230 |
55 |
0.1418 |
0.5326 |
-0.0653 |
0.6048 |
-0.2127 |
-0.0626 |
0.5313 |
9.285 |
17.5 |
0.0255 |
60 |
0.1732 |
0.4571 |
0.0861 |
0.5530 |
-0.1064 |
0.0650 |
0.4676 |
6.788 |
14.5 |
0.0335 |
65 |
0.2191 |
0.3779 |
0.2493 |
0.4918 |
0.0163 |
0.2124 |
0.3954 |
4.631 |
11.7 |
0.0502 |
70 |
0.2802 |
0.2951 |
0.4354 |
0.4119 |
0.1781 |
0.4066 |
0.3072 |
2.874 |
9.4 |
0.0718 |
75 |
0.3586 |
0.2124 |
0.6553 |
0.3178 |
0.3819 |
0.6513 |
0.2137 |
1.572 |
7.4 |
0.1013 |
80 |
0.4475 |
0.1362 |
0.9235 |
0.2173 |
0.6408 |
0.9622 |
0.1274 |
0.719 |
5.6 |
0.1480 |
85 |
#N/A |
0.0753 |
1.2542 |
0.1201 |
0.9959 |
1.3887 |
0.0586 |
0.255 |
4.3 |
0.2097 |
The logit transformations of the proportions surviving appear in column 4 of Table 6. For example, the logit transformation of the l20 is calculated as follows:
The logit transformation of the conditional life table for males based on the AIDS life table with e0=50 in column 5 of Table 6 appears in column 6 of Table 6. As can be seen from Figure 2, the AIDS model does not fit the data particularly well, but fits better than any table which does not reflect the impact of HIV on mortality.
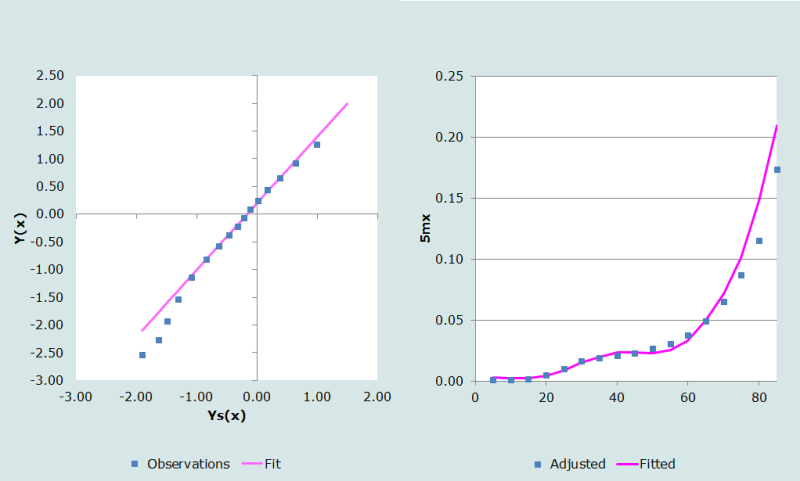
The coefficients, α and β are determined as the intercept and slope of the straight line fitted to the logit transformations in columns 4 and 6 of Table 6 over the range of ages chosen by the user (45 and 80 in this example), namely 0.1928 and 1.2008 respectively.
These coefficients are then applied to the logit transformation of the conditional model life table to produce the fitted logits in column 7 of Table 6. Thus, for example, the fitted logit at age 20 is calculated as follows:
These values are then used to produce the fitted life table in column 8 of Table 6. For example the value at age 20 is calculated as follows:
The conditional years of life lived, Tx, which appear in column 9 of Table 6, are then calculated from the fitted life table. These numbers are then used to produce the smoothed mortality rates which appear in column 10 of Table 6. For example, for age 80
The life expectancies which appear in column10 of Table 6 are the numbers in column 9 divided by the numbers in column 8. For example, the life expectancy at age 65 is
Diagnostics, analysis and interpretation
Checks and validation
The estimate of completeness is 94 per cent. The first check on this result is a comparison with the results for the opposite sex. For example, applying the same method as described above for men to the data for women during the same period (in the SEG_South Africa_females workbook) gives an estimate of completeness of 93 per cent. Past research (Dorrington, Moultrie and Timæus 2004) leads to the expectation that the estimates should be similar, so the results are sufficiently close as to validate the estimates.
A second check on the results is to compare them with the result from the Generalized Growth Balance method (in the GGB_South Africa_males workbook), which estimated the completeness of death reporting over the age range 5 to 84 to be 92 per cent. This again supports the result.
A third check is to compare estimates of various key indicators of mortality with those from other sources, such as previous estimates for the country or the World Population Prospects (UN Population Division 2011). The estimate of 45q15 from the observed mortality rates after adjusting for incompleteness is 51.9 per cent, while the estimate of 45q15 from the WPP for the period 2000-2005 is 52.9 per cent, again suggesting no reason to question the results.
As a matter of interest, application of the Preston and Coale method to these data (estimating the population in the middle of the period as that average of the two survey populations) provides an estimate of completeness, using the same age range, of 84 per cent. Increasing the minimum age of range of the data used to determine delta to 35 increases the estimate to 86 per cent, still somewhat lower than the estimate of 94 per cent produced above.
Interpretation
Inspection of the estimates of completeness (Figure 1) suggests that the completeness of death reporting appears to fall steadily with age from about age 55, which is consistent with migrant workers retiring from urban areas to rural areas, where completeness of registration was lower. Since these estimates were produced using the estimate of delta produced by application of the Generalized Growth Balance method and it is entirely plausible that people have retired from urban to rural areas, reducing delta to produce a more level set of estimates is inappropriate.
Since migration has been taken into account, the falloff in estimates of completeness at the younger ages is probably due to the opposite of what is happening at the older ages, namely, young people moving from rural to urban areas to find employment. It would be wrong, therefore, to allow estimates at these ages to influence the estimate of overall completeness unduly.
The lack of smoothness in the series of the ratios of is determined by the estimates of the population from the census and survey, and not from the deaths. Thus, the erratic nature of this series is probably indicative of errors due to relative undercounting in particular age groups and/or age misreporting in the census or survey population estimates.
Method-specific issues with interpretation
Source of reported deaths
Generally there are two sorts of problems with the death data: those that lead to under/over coverage that is constant by age, which is precisely what the method is intended to address, and those which lead to differential coverage by age, which can distort the estimates. Although the general approach remains essentially the same irrespective of the source of the death data, different sources of death data are prone to different biases which might impact on the interpretation of the results. These are illustrated by way of particular examples, but, in general terms, the analyst needs to look out for the following biases in the death data.
(i) Vital registration
If the proportionate split of the population between urban and rural (or appropriate proxies) areas differs significantly by age and the completeness of reporting of deaths in urban areas is significantly higher than it is in rural areas, then the assumption that completeness is independent of age is likely to be violated by a falling off of completeness with age at ages over 50 if a proportion of people move from urban to rural areas on retirement. If ignored, this violation is likely to lead to an underestimate of the average level of completeness.
(ii) Deaths reported by households
The data are subject to four potential problems:
- If a significant proportion of households dissolve on the death of a key person (e.g. the sole breadwinner), then the deaths of such people go unreported, leading to a violation of the assumption that completeness is invariant with age. If a significant proportion of deaths in some age groups are of individuals who do not live in private households (for example, they live in homes for the elderly), the breach of the assumption could be even more severe. However, this is not an issue in most developing countries.
- In situations where young adults leave the home they grew up in to work in urban areas, it is possible that they are regarded as being members of more than one household (or of neither household) and their deaths could be reported more than once (or not at all), again leading to a violation of the assumption of constant reporting of deaths by age. In this case, one can limit the impact by ignoring the data below a specific age in determining completeness.
- Reference period error: Since there is often confusion about the exact period for which deaths are to be reported, not to mention uncertainty about exact dates of death, it is possible for there to be overall under- or over-reporting of deaths. Provided one can assume that this is independent of the age of the deceased, this distortion will be accounted for in the estimate of completeness and is not a problem for estimating mortality rates.
- The reference period covers a small proportion of the intercensal period, for example, the common situation in which households report on deaths for the year preceding the census. Not only might such a short period result in significant random fluctuation, but in addition one does not have an estimate of the population at the start of this reference period. How one might deal with this is illustrated in the examples given. Essentially, if one has, in addition, deaths reported by households at the first census, one can use the two sets of data on deaths to estimate the number of deaths during the intercensal period, as was discussed above. However, since the question asking households to report on deaths in the previous year was used relatively seldom before the 2010 round of censuses, one may only have the single set of data on deaths. In this case, provided there are no reasons for assuming that the age pattern of mortality has changed rapidly over the period, it is recommended that one calculates the age-specific death rates for the year and applies these to the person-years of life lived for the interval to get an estimate of deaths for the period. If there are reasons for suspecting that mortality has changed rapidly, for example due to HIV/AIDS, then this adaptation is likely to underestimate or overestimate the mortality and the use of death distribution methods is not recommended.
(iii) Deaths recorded in health facilities
Little is known about how well this source of data works. However, it can be expected that completeness would depend on the distribution of health services from which the data have been gathered, and in many developing countries such services are likely to be concentrated in urban areas. So, again, if the proportion of the population living in urban rather than rural areas varies with age, then completeness cannot be assumed to be independent of age. It is also possible that certain causes will predominate in facilities, and if these causes are significant and age-related, this could lead to a further violation of the assumption of constant completeness by age.
In all such cases, one should avoid the temptation of adjusting delta to produce a level sequence of the ratios, and ensure that the estimate of c is determined over an age range which excludes the ages where distortions exist.
General diagnostic interpretation
In practice both the sequences of and are affected by violations of the assumptions. However, part of the power of this technique is that most of the typical violations of assumptions produce fairly distinctive characteristic deviations from the expected horizontal plot and in certain circumstances these patterns are interpretable. The following are examples:
(a) Incorrect estimate of relative coverage of the censuses: If δ is too high the sequences fall nearly linearly with increasing age towards the underlying value of completeness and vice versa, as can be concluded from inspection of equation (1) below. The effect is greater for than for .
(b) Exaggeration of reported age: Typically, relatives reporting deaths exaggerate the person’s age at death more than living individuals reporting their own ages. This produces rising sequences of points which are imperceptible up to the age at which exaggeration begins, followed by a sharp upward curve thereafter. Again, it can be seen from inspection of Equation 1 below, that age exaggeration leads to an increase in the number of deaths in the older age categories. In addition, transfers within an age category lead to those deaths being multiplied by a larger exponential term, although this effect is far smaller. Although such a pattern would also be produced by rising completeness in death registration with age beyond a certain age there appears to be no evidence of this in practice (Preston, Coale, Trussell et al. 1980).
(c) Age misstatement in the population estimates and age-specific miscounting: This is exhibited by an erratic sequence of the ratios over the age span. Sinceis cumulative in form, it tends to follow the age distribution of the population quite closely and hence, if there are zigzags, it is likely that the peaks are associated with inflated population estimates and the troughs with deflated ones. If these fluctuations are independent of age they will not distort the estimate of completeness particularly. However, if they are systematic, for example due to unaccounted for migration beneath a certain age, it may be desirable not to include these points in estimating the completeness.
Examples using deaths reported by households in a census/survey
The examples below use the same data as used in the SEG_South Africa_males and SEG_South Africa_females workbooks with the exception that instead of using the vital registration as the source of the death data, deaths are estimated from deaths reported by households in the 2001 census and the 2007 Community survey as having occurred in the year preceding the census/survey. These numbers are given in Table 7.
Table 7 Deaths reported by households to have occurred in the year preceding census/survey, South Africa
|
2001 Census |
2007 Community Survey |
||
Age |
Males |
Females |
Males |
Females |
0-4 |
35,873 |
32,096 |
48,322 |
44,418 |
5-9 |
3,868 |
3,155 |
4,505 |
5,216 |
10-14 |
2,590 |
2,284 |
3,442 |
3,259 |
15-19 |
5,628 |
5,122 |
8,246 |
7,878 |
20-24 |
10,976 |
13,246 |
16,360 |
21,702 |
25-29 |
17,787 |
19,727 |
27,551 |
35,840 |
30-34 |
20,038 |
18,292 |
34,832 |
42,576 |
35-39 |
19,816 |
15,521 |
38,061 |
34,809 |
40-44 |
17,417 |
12,124 |
33,604 |
28,823 |
45-49 |
15,840 |
10,105 |
27,829 |
20,973 |
50-54 |
15,077 |
9,144 |
28,223 |
18,891 |
55-59 |
12,781 |
7,755 |
22,868 |
13,118 |
60-64 |
13,428 |
10,367 |
18,775 |
14,912 |
65-69 |
11,820 |
10,195 |
17,532 |
14,298 |
70-74 |
11,885 |
10,809 |
14,879 |
14,645 |
75-79 |
8,794 |
8,393 |
12,966 |
14,151 |
80-84 |
7,484 |
9,371 |
9,204 |
12,063 |
85+ |
7,115 |
12,389 |
11,735 |
18,178 |
The numbers of deaths occurring between the date of the Census (midnight between 9 and 10 October 2001) and the survey (assumed to be midnight between 14 and 15 February 2007) are estimated using the Estimating deaths_South Africa_males_hhd and the Estimating deaths_South Africa_females_hhd workbooks.
Applying the Synthetic Extinct Generations method to these data for males using the estimate of relative incompleteness of census coverage (delta) derived from the application of the Generalized Growth Balance method to these data, SEG_South Africa_males_hhd, suggests that these estimates of the number of deaths are more or less as completely reported as the vital registration. However these data estimate 45q15 at 53.9 per cent, which although similar, is slightly higher than the estimate produced using registered deaths. Applying the Synthetic Extinct Generations method to these data for females, SEG_South Africa_females_hhd, suggests that the deaths of women reported by households are far less complete than the registered deaths and estimates 45q15 at 49.3 per cent. This is higher (and less plausible relative to the probability for males) than the 42 per cent produced using registered deaths.
The reason for the much poorer performance of the method applied to deaths of women reported by households can be seen by a comparison of the estimated numbers of deaths for the period derived from deaths reported by households to the numbers expected after correcting the vital registration for incompleteness of reporting, as shown in Table 8. From this we see that there is a significant decline in completeness of reporting of deaths of women by households with age from age 55, probably as the result disintegration of households on the death of these women, usually because these households were headed by the women who died.
There is also evidence of over-reporting of deaths below age 30 for males and 25 for females.
Table 8 Ratio of estimates of deaths derived from deaths reported by households to the expected numbers of deaths, South Africa
|
Males |
|
Females |
|
||
Age |
Reported |
Expected |
Ratio |
Reported |
Expected |
Ratio |
0-4 |
|
|
|
|
|
|
5-9 |
22,683 |
16,979 |
134% |
22,995 |
14,575 |
158% |
10-14 |
16,462 |
12,224 |
135% |
15,173 |
10,349 |
147% |
15-19 |
38,013 |
27,784 |
137% |
35,666 |
26,874 |
133% |
20-24 |
74,934 |
59,946 |
125% |
95,993 |
84,611 |
113% |
25-29 |
124,403 |
112,129 |
111% |
152,718 |
154,437 |
99% |
30-34 |
150,792 |
158,796 |
95% |
166,488 |
170,680 |
98% |
35-39 |
159,016 |
159,137 |
100% |
137,837 |
141,399 |
97% |
40-44 |
140,172 |
148,269 |
95% |
111,910 |
115,746 |
97% |
45-49 |
120,016 |
131,988 |
91% |
85,284 |
93,408 |
91% |
50-54 |
118,989 |
121,242 |
98% |
76,941 |
81,793 |
94% |
55-59 |
97,977 |
105,641 |
93% |
57,353 |
72,131 |
80% |
60-64 |
88,088 |
98,089 |
90% |
69,220 |
78,877 |
88% |
65-69 |
80,451 |
90,359 |
89% |
67,007 |
86,099 |
78% |
70-74 |
72,827 |
79,663 |
91% |
69,536 |
93,404 |
74% |
75-79 |
59,632 |
69,665 |
86% |
61,942 |
88,314 |
70% |
80-84 |
45,365 |
52,533 |
86% |
58,410 |
77,084 |
76% |
85+ |
51,779 |
50,387 |
103% |
83,753 |
108,002 |
78% |
In the situation where only the most recent census asked about deaths in the previous year, the number of deaths in each age group between the times of the 2001 Census and the 2007 Community Survey using only the deaths reported by households in the 2007 Community Survey are estimated as follows:
Applying the method to these estimates of the deaths produce estimates of 45q15 of 58.1 per cent for males and 55.6 per cent for females. Unlike the previous estimates, these are estimates of mortality in the year preceding the second census/survey and therefore might be expected to be higher than those for the whole period, since mortality has been increasing over the period due to HIV/AIDS. However, as might also be expected, deriving an estimate from a single year of deaths (derived, in addition, in this case from a relatively small sample survey) produces far less reliable estimates, particularly in the case (for these data) of females. Alternative estimates (Bradshaw, Dorrington and Laubscher 2012) suggest that for 2006 the correct probabilities should be closer to 55 per cent for males and 45 per cent for females.
Detailed description of method
Mathematical exposition
Referring to this method as the Synthetic Extinct Generations is somewhat misleading, since it is not, as was the case with the method proposed by Preston and Coale, et al (1980), derived from the idea of extinct generations originally proposed by Vincent (1951).
Instead the method relies on a reconstruction of the past rather than projecting the future. The generalised form of this reconstruction is outlined in the seminal paper by Preston and Coale (1982). In order to derive the expression for N(y,t), the population at time t aged y exactly, in terms of deaths and growth rates at time t, we start with an expression for the number of deaths at age x>y and ignore migration for simplicity. (Migration can be included simply by deducting the rate of net immigration (i.e. immigration less emigration) from the growth rate. That is replacing r(a,t) by r(a,t)- i(a,t) where i(a,t) represents the rate of net immigration at age a at time t).
or
Thus, it is possible to derive an estimate of the population at each age based on the age-specific numbers of deaths over a particular interval and the age-specific population growth rates. Comparison of these estimates of the population to the estimates derived from censuses gives an estimate of completeness of the deaths relative to that population.
Implementation of the method in practice
Since the data one typically works with are subject to age misstatement, in practice one usually works with data grouped into five-year age groups.
Thus, assume that in practice one has data on the following: the number of reported deaths over a number of years, from times t1 to t2, in five-year age groups, , up to an open interval at age A,
; and the number of people in the population at each of times t1 and t2, in the same age groups reported by the censuses, up to (where t = either t1 or t2). These data can then be used to apply the method by computing< and , and approximating by
and
by
As suggested by Bennett and Horiuchi (1981), a computational form of Equation 1 can be derived, namely that
where represents the number of people in the population who turned x between the census at time t1 and the census at time t2, and represents the average annual growth rate of the population aged x to x + 4 last birthday between times t1 and t2.
Bennett and Horiuchi (1981, 1984) suggest using the age group-specific growth rates to improve both the at the older ages and , where A is the age at the start of the open-ended age interval. They suggest calculating as follows:
where represents the reported deaths aged A and older, and represents life expectancy at age A.
They also suggest that in order to allow for the curvature, particularly at the older ages, Equation 2 could be modified as follows:
where
In addition to this, they suggest that over the age of 60 the be approximated by “imposing a stable population curve over the five-year span and then determining the area under the curve accordingly” (Bennett and Horiuchi 1981: 210). However, in practice the data are rarely accurate enough to warrant such a refinement, and it seldom makes much difference to the estimate of completeness.
In practice, in order to correct, to some extent, for the effects of digit preference in age reporting and also to make the death data consistent with population data for conventional five-year age groups, it is usual to compute . Furthermore, since the sequence of ratios (or even as suggested by Bennett and Horiuchi (1981)) is usually still somewhat erratic because of age misreporting and differential omission of persons in particular age spans, it is usual to assume that the percentage reported is roughly constant with respect to age for ages greater than, say, 10. One estimates this fixed proportion, c, by either the mean or median of the values of over a representative span of ages (after, if necessary, correcting the age group-specific growth rates for the differential completeness of the two censuses). Allowing for differential completeness of the two censuses is achieved by adding a constant factor, , to the age group-specific growth rates derived from the reported population numbers to produce a ‘flat sequence’ of .
To see this, suppose and . Then
where
Thus , where t is the length of the intercensal period, gives an indication of the differential completeness between the two estimates of the population used to estimate the age group specific growth rates.
The sequence of values is usually plotted together with that of , where. The latter ratio tends to be more stable and assists with interpretation of the data.
Extension
If the ages were recorded accurately and the assumption of constant census coverage by age held (not a very likely situation), then the method could be adapted to deal with the situation where completeness of reporting of the deaths was constant only for a limited age range (x to x + n) by applying a truncated version of the method which eliminates from consideration deaths and population aged x + n and older. This adaptation could, for example, be applied to vital registration data where completeness might fall off above retirement age if people retired from urban to rural areas. It could also be applied where deaths reported by households where household might disintegrate on the death of the last adult. However, unless x+n is high this method is unlikely to be very robust. Analogous adaptation of the Generalized Growth Balance method is easier and seems to be a little more robust.
Further reading and references
Analysis of the sensitivity of the method to common data errors and violation of the assumptions is fairly limited. However, the reader is referred to Hill, You and Choi (2009) for an analysis of the assumptions underlying the death distribution methods in the absence of HIV and to Dorrington and Timæus (2008) for an analysis in a population experiencing significant HIV. Murray, Rajaratnam, Marcus et al. (2010), in contrast, used stochastic simulations to assess these methods. They concluded, with perhaps unrealistic assumptions about migration, that the methods were not particularly reliable.
Bennett NG and S Horiuchi. 1981. "Estimating the completeness of death registration in a closed population", Population Index 47(2):207-221. doi: https://dx.doi.org/10.2307/2736447
Bennett NG and S Horiuchi. 1984. "Mortality estimation from registered deaths in less developed countries", Demography 21(2):217-233. doi: https://dx.doi.org/10.2307/2061041
Blacker J. 1988. An Evaluation of the Pakistan Demographic Survey. Karachi: Pakistan Federal Bureau of Statistics. https://dx.doi.org/10.2307/2061041
Bradshaw D, RE Dorrington and R Laubscher. 2012. Rapid Mortality Surveillance Report 2011. Cape Town: South African Medical Research Council. https://www.samrc.ac.za/sites/default/files/attachments/2022-08/RapidMortality2011.pdf
Dorrington RE, TA Moultrie and IM Timæus. 2004. Estimation of mortality using the South African 2001 census data. Monograph 11. Centre for Actuarial Research, University of Cape Town. https://blogs.lshtm.ac.uk/iantimaeus/files/2024/03/Dorrington-Moultrie-Timaeus-Mono11.pdf
Dorrington RE and IM Timæus. 2008. "Death Distribution Methods for Estimating Adult Mortality: Sensitivity Analysis with Simulated Data Errors, Revisited," Paper presented at Population Association of America 2008 Annual Meeting. New Orleans, Louisiana, 17-19 April.
Hill K, D You and Y Choi. 2009. "Death distribution methods for estimating adult mortality: Sensitivity analysis with simulated data error", Demographic Research 21(Article 9):235-254. doi: https://dx.doi.org/10.4054/DemRes.2009.21.9
Murray CJL, JK Rajaratnam, J Marcus, T Laakso and AD Lopez. 2010. "What can we conclude from death registration? Improved methods for evaluating completeness", PLoS Med 7(4):e1000262. doi: https://dx.doi.org/10.1371/journal.pmed.1000262
Preston SH, AJ Coale, J Trussell and M Weinstein. 1980. "Estimating the completeness of reporting of adult deaths in populations that are approximately stable", Population Index 46:179-202. doi: https://dx.doi.org/10.2307/2736122
Timæus IM. 2007. "Impact of HIV on mortality in Southern Africa: Evidence from demographic surveillance", in Caraël M and JR Glynn (eds). HIV, Resurgent Infections and Population Change in Africa Springer, pp 229–243. doi: https://dx.doi.org/10.1007/978-1-4020-6174-5_12
UN Population Division. 2011. World Population Prospects: The 2010 Revision, Volume I: Comprehensive Tables. New York: United Nations, Department of Economic and Social Affairs, ST/ESA/SER.A/313. https://www.un.org/development/desa/pd/sites/www.un.org.development.desa.pd/files/files/documents/2020/Jan/un_2010_world_population_prospects-2010_revision_volume-i_comprehensive-tables.pdf
Vincent P. 1951. "La mortalite des vieillards", Population 6:182-204. doi: https://dx.doi.org/10.2307/1524149
- Printer-friendly version
- Log in to post comments